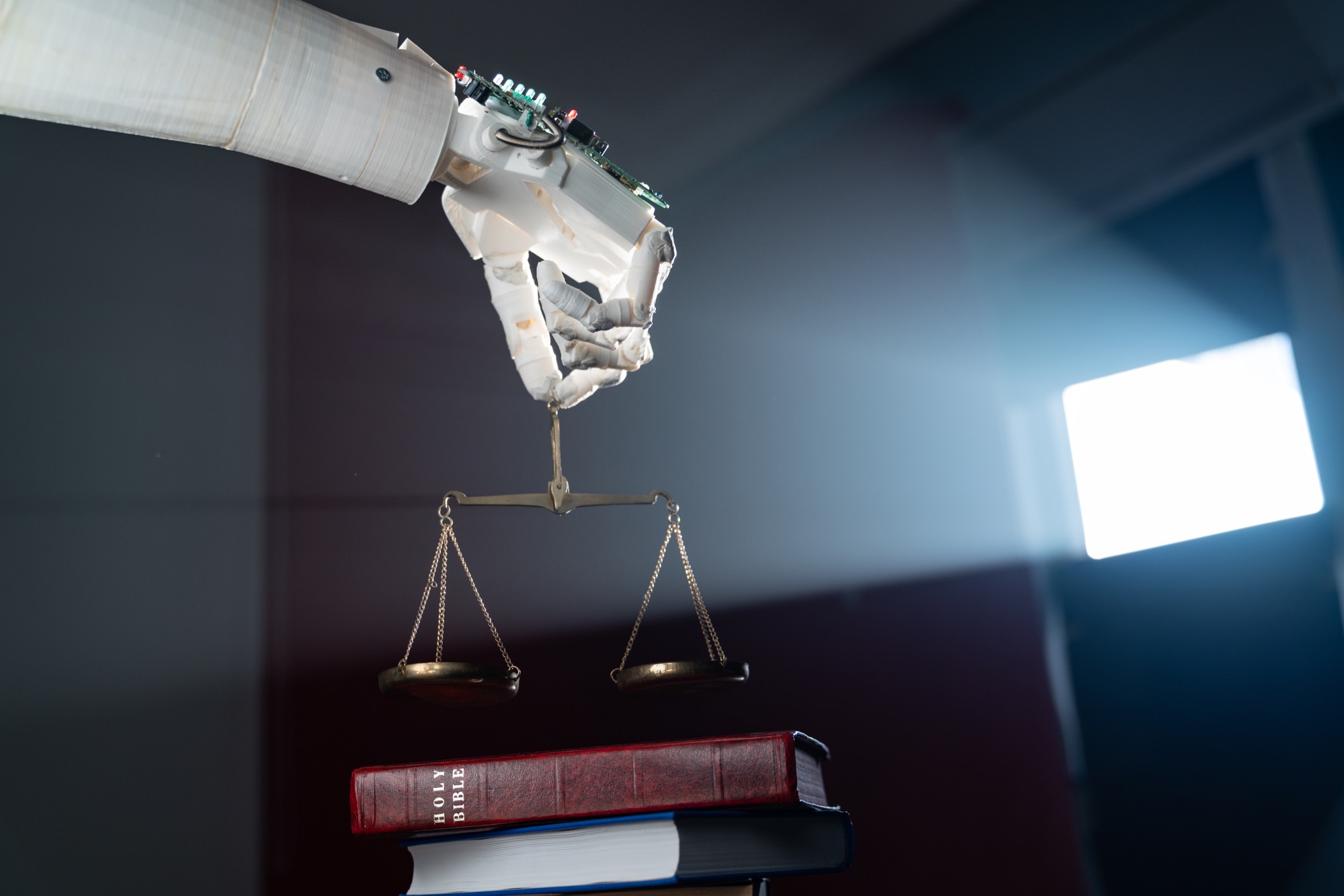
While healthcare algorithms can improve prediction and diagnosis, they often “bake in” biases that exist in the healthcare system and its data. We develop methods to expose bias in existing predictive models that are used to risk-stratify millions of patients for clinical or policy purposes. We have also developed methods to identify mechanisms of bias in clinical care. Finally, we investigate how to mitigate bias through cutting-edge statistical techniques and incorporating novel data, including socioeconomic or patient-generated health data, with standard features. Our goal is to be able to perform “equity audits” of common healthcare algorithms in order to create more equitable, fair deployment of AI.